The Advent of the AI Age in Healthcare
While OpenAI’s introduction of ChatGPT to the public in late 2022 solidified AI’s place in the limelight for its uses in healthcare and beyond, the proponents of AI for our healthcare system have been making steady strides in the background for many years before. At Putnam, we have been supporting clients over the past 5+ years explore the opportunities and challenges of AI, continuously evolving our thinking to keep with the pace of change, and often validating outcomes through other means of research.
In recent years, we have seen continued expansion of development interests spanning possible use cases from drug discovery to patient interaction. This is also true for patient diagnosis and monitoring, marking an explosion in the numbers of experimental academic studies as well as actual clinical pilots and adoption.
In this article, we will provide a perspective on the opportunities and barriers brought about by AI in diagnosis and monitoring from a biopharmaceutical industry lens.
The Challenge
Timely diagnosis and treatment is an ongoing issue, with rare disease and health equity as factors exacerbating these challenges.
Roughly 300 million patients live with rare diseases globally across more than 7,000 diseases, and ~95% of these rare diseases do not have an approved treatment1.
Although, even where there is an approved treatment, the ability to find diagnosed patients can still be a complicated challenge. According a 2022 study by the EveryLife Foundation for Rare Diseases, it takes more than 6 years and nearly 17 doctor visits, hospitalizations, and other health-related trips, to receive a rare disease diagnosis after symptoms begin2.
There are many reasons that can be contribute to the complex patient journey of a rare disease patient before they land in the care of the right experts, including:
- Early symptoms are not easily recognized or suspected.
- Lack of guidelines or protocols in place to effectively screen patients.
- Complex imaging– e.g. physicians only having access to common devices, or physicians not having access to the imaging experts with the right level of expertise.
- Potential lack of easy access to genetic testing / genetic counselors (for genetic diseases).
The same challenge can also plague appropriate use of treatments. A 2020 study exploring under/overtreatment definitions in oncology found 256 articles with the terms under or overtreatment, with 236 articles using the term undertreatment3.
The rising conversations around health equity also come into play here, with variety of biases having been brought to light after the implicit bias phenomenon was recognized. The 2017 review (BMC Med Ethics 20173) identified 35 articles that found implicit bias in healthcare professionals – with a significant positive relationship between level of implicit bias and lower quality of care. Other examples include:
- Treatment decision biases – e.g. women with chronic pain are less likely to receive pain medication (Harvard Health 20174)
- Algorithmic biases – A recent study by the US Agency for Healthcare Research and Quality (JAMA Health Forum 20235) suggested that treatment algorithms are in widespread use and may be biased. In 2021, the Maternal Fetal Medicine Network removed race as a risk factor for poor outcomes in the VBAC algorithm. Now, white people with chronic hypertension are more educated about their risk of complicated delivery and African American and Hispanic people undergo fewer unnecessary c-sections (Nature Medicine 20216).
AI as a Part of the Solution
AI is emerging as a more meaningful solution in diagnostics.
Although AI has been used in supporting diagnostics for several years, there have been an exponentially increasing number of developments in using AI for diagnosis and patient monitoring, with examples spanning:
Breast Cancer: AI for staging and mammograms
- Staging: Assessing the extent of cancer spread by histopathological analysis of sentinel axillary lymph nodes (SLNs) is an important part of breast cancer staging. The Cancer Metastases in Lymph Nodes Challenge 2016 (CAMELYON16) investigated the potential of machine learning algorithms for detection of metastases in SLN slides. 7 of these deep learning algorithms showed greater discrimination than a panel of 11 pathologists in a simulated time-constrained diagnostic setting, with an area under the curve of 0.994 (best algorithm) vs 0.884 (best pathologist)7
- AI Assisted Mammograms: AI assisted mammograms have now become increasingly commonplace. In fact, recent news coverage has shifted from the sensitivity of AI testing to titles that read: “Mammography AI can cost patients extra. Is it worth it?” (CNN, 20248)
- AI-read Mammograms: Preliminary results from a Swedish study (Lancet, 20239) show the potential for AI to read mammograms alone. The study recruited 80,000 women split into 2 groups: AI-read mammograms vs. double-read (non-AI) mammograms showed AI supported mammogram readings had a similar cancer detection rate as the double-read mammograms, with a substantially lower screen-reading workload.
Diabetic Retinopathy: AI as a reimbursed diagnostic tool
- In 2016, the article “Development and validation of a deep learning algorithm for detection of diabetic retinopathy in retinal fundus photographs,” by Gulshan et al and the Google team (JAMA 201610) showed 90+% accuracy in detecting diabetic retinopathy (DR) from color fundus photos, proving the possibility of using AI in diagnosing retinal diseases.
- By 2018, the FDA11 approved IDx-DR, the first medical device to use artificial intelligence to detect greater than a mild level of the eye disease diabetic retinopathy in adults who have diabetes.
- By 2021, the US CMS introduced12 a new ophthalmology CPT code, 92229, the first CPT code covering reimbursement for this new automated technology.
- In 2024, the FDA approved AEYE-DS13 for diabetic retinopathy, the first point-of-care AI screening with a handheld device.
Medical LLM: ChatGPT vs. Med-Gemini – Is Medical LLM in the cards?
-
- While still in the experimental phase, it is important to note the significant limitations of LLMs, which we expect will continue, making it critical to see them as a supplemental tool.
- In 2024, Google released a paper14 on Med-Gemini, a new family of highly capable multimodal models built on top of their powerful Gemini models. They highlighted the potential of AI in supplementing many of the duties that HCPs conduct today. Over fifty-eight pages long, many examples were shown such as:
- Diagnosis of a patient via dialogue
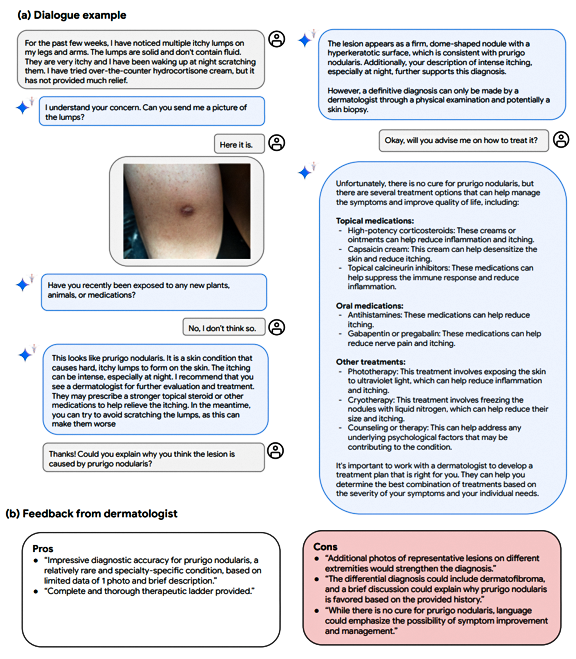
- Summarizing of EHR data
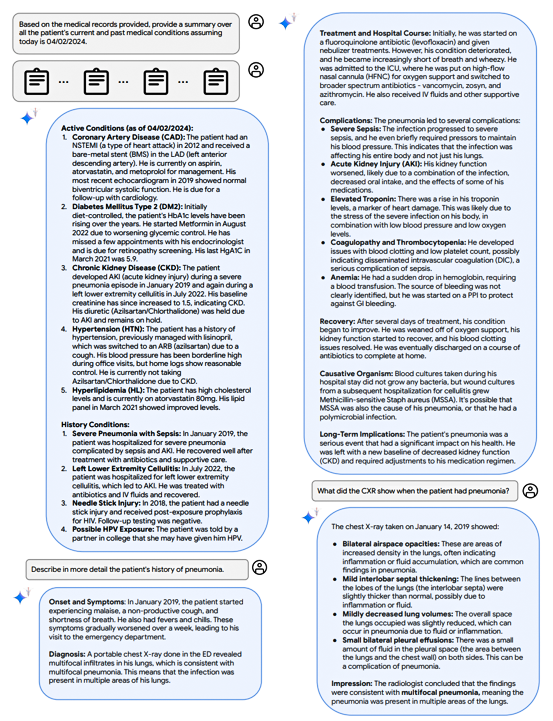
Opportunities from AI
We see AI as yielding opportunities for biopharmaceutical manufacturers.
The increasing value proposition of AI in improving diagnosis and appropriate treatment can potentially yield significant benefit to both patients and manufacturers, a win-win result.
In diseases where patients are hard to find, AI has a potential to assist physicians (particularly non-experts) in earlier diagnosis. For therapeutic areas with complex treatment algorithms, AI could help reduce the barrier to regular risk assessments/staging activities.
While the experimental applications of AI continue to be explored by academia, biopharmaceutical manufacturers are in a unique position to directly support these developments, particularly in areas of rare disease where there may not other be sufficient academic interest. Additionally, for areas of algorithmic or unintended bias, AI has the potential to be trained without these implicit biases.
Caution and Considerations
Despite the promise, caution is critical. Investments in AI should be treated like investments in other assets. Many AI solutions are currently still in the experimental phase. Similar to drug development/investment, care and strategy is needed in AI investments. While AI can provide opportunities, it comes with its own set of challenges, such as the risk of amplifying biases that the AI has been trained on15.
It is important ask and explore strategic questions such as:
- Where are the opportunities in the patient journey?
- What is the true problem statement(s) to tackle?
- How big is this opportunity?
- What are the requirements of the AI solution to unlock this opportunity?
- What are the requirements needed for technical feasibility in conducting an AI study?
- Can sufficient data be sourced?
- What is needed to optimize the AI study design?
- What are the right variables to focus on?
- Inclusion exclusion criteria?
- What are the implementation considerations for successful pull-?
All in all, the continued progress in AI development shows that there can be feasible roadmaps to success in these investments. As such, biopharmaceutical manufacturers should not ignore this potential avenue as they weigh their strategic options for the future.
Sources:
- The Lancet. (2024). The landscape for rare diseases in 2024, 12(3), E341. [Online]. Available from: Link
- EveryLife Foundation for Rare Diseases. (n.d.). Delayed Diagnosis Study. [Online]. Available from: Link
- Smedley, B. D., Stith, A. Y., & Nelson, A. R. (Eds.). (2003). Implicit bias in healthcare professionals: a systematic review. BMC Medical Ethics, 18, 19. [Online]. Available from: Link
- Women and pain: Disparities in experience and treatment. (n.d.). Harvard Health.
- JAMA Health Forum. (2023). Awareness of Racial and Ethnic Bias and Potential Solutions to Address Bias With Use of Health Care Algorithms, 4(6), e231197. [Online]. Available from: Link
- Stanford Medicine. (2023, October 20). AI, medicine, and race: Why ending structural racism in healthcare now is crucial. Scope Blog. [Online]. Available from: Link
- Esteva, A., Kuprel, B., Novoa, R. A., Ko, J., Swetter, S. M., Blau, H. M., & Thrun, S. (2017). Diagnostic assessment of deep learning algorithms for detection of lymph node metastases in women with breast cancer. JAMA, 318(22), 2199-2210. [Online]. Available from: Link
- Christensen, J. (2020, February 12). Mammography AI can cost patients extra. Is it worth it? CNN. [Online]. Available from: NA
- Houssami, N., Noguchi, M., & Evans, G. (2023). Artificial intelligence-supported screen reading versus standard double reading in the Mammography Screening with Artificial Intelligence trial (MASAI): a clinical safety analysis of a randomised, controlled, non-inferiority, single-blinded, screening accuracy study. The Lancet, 24(8), P936-P944. [Online]. Available from: Link
- Gulshan, V., Peng, L., Coram, M., Stumpe, M. C., Wu, D., Narayanaswamy, A., … & Webster, D. R. (2016). Development and validation of a deep learning algorithm for detection of diabetic retinopathy in retinal fundus photographs. JAMA, 316(22), 2402-2410. [Online]. Available from: Link
- S. Food and Drug Administration. (2018, April 11). FDA permits marketing of artificial intelligence-based device to detect certain diabetes-related eye problems. [Online].
- Federal Register. (2021, July 9). 2021-14973.pdf. [Online]. Available from: Link
- Notal Vision. (n.d.). FDA clears first fully autonomous AI for portable diabetic retinopathy screening. PR Newswire. [Online]. Available from: Link
- Carreira-Perpinan, M. A., & Zisserman, A. (2024). Res2Net: A new multi-scale backbone architecture. arXiv preprint arXiv:2404.18416. [Online]. Available from: Link
- Pinto, A. M., & Schook, L. B. (2021). Toward precision medicine: A new biomedical framework for the 21st century. Nature Medicine, 27(12), 2176-2182. [Online]. Available from: Link